The Importance of Predicting Drug Response
In cancer treatment, time is of critical importance.
According to the American Cancer Society, an estimated 42% of all cancer cases in the U.S., as well as roughly 45% of cancer deaths, can be directly attributed to risk factors that could have been changed had the patient known about their status in time. This includes risks such as smoking, sun exposure, genetic factors and more. As a result, early detection and cancer screening tests have been shown to prevent thousands of additional cancer cases and deaths each year.
But that’s not all. Once a patient has been diagnosed, the clock starts ticking. Their doctors are then in a race against time to treat their disease before it advances so far that they can no longer fight it.
This is why predicting drug response has become so important. When physicians know what types of drugs will work for their patients, they’re better able to make the correct treatment decision the first time, saving precious time that would otherwise be wasted trying and failing with other plans.
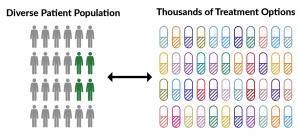
The ability to accurately predict drug response enables patients to be matched with precision treatment options.
There are business considerations at play here as well. Rather than cycling through multiple courses of treatment, and billing the patient’s insurer for each one, an accurate drug response prediction the first time helps payers and healthcare systems save on their own costs.
Overview of Current Methods
Given all of these advantages, it’s a shame that the current methods of prediction leave so much to be desired. As it stands, there are three primary ways to predict drug response: PD-L1, DNA mutations, and RNA fusions.
PD-L1 (Programmed death-ligand 1) is a protein that plays a role in the immune system, expressing in response to a range of disease state events. In short, when your body is fighting a disease, PD-L1 fires off in response. However, as a predictive biomarker, PD-L1 has its limitations. Although it has been shown to be effective in some cases, researchers have found that the protein only accurately responds in less than 30% of cancer cases, due to the uneven immune system response.
DNA mutations are another popular method of predicting drug response, due to the fact that cancer is caused by genetic mutations and alterations. But using these mutations as a way to predict drug response is difficult due to the fact that every case is different and the mutations that lead to a specific diagnosis in one patient may have an entirely different result in another patient.
RNA fusions are another prediction tool that falls victim to the variables of biology. Although RNA analysis is an emerging and powerful tool in diagnostics, detecting the individual fusions that arise during the progression of disease can lead to false negative results based on everything from poor RNA samples, difficulty detecting low level gene fusions, and more.
The fact is, single analyte biomarkers have failed us.
Multidimensional Biomarkers for Predicting Drug Response
However, by taking a broader, more multidimensional view, researchers can better predict drug responses. This includes everything from looking at multiple analytes, leveraging machine learning to improve data analysis, using negative and positive predictive values to predict responses based on statistical norms, and considering variables such as specificity and sensitivity.
This is exactly the approach we have taken in building our Health Expression Models, using machine learning to define these multigenic gene expression models.
We built these models by isolating purified immune cell populations, utilizing whole-transcriptome sequencing to evaluate the global transcriptome profile of the immune cell, and then evaluating the gene expression signals using machine learning to identify the genes whose expression levels uniquely define each immune cell. We pair these models with other RNA data using Predictive Immune Modeling to generate some of the most powerful multidimensional biomarkers available today.
Interested in seeing the true power of multidimensional biomarkers for predicting drug response? Contact us today.