Machine Learning and Precision Diagnostics
By Tiange (Alex) Cui, Ph.D.
The term ‘Machine Learning’ was coined in 1959 by Arthur Samuel, who defined it as — “The field of study that gives computers the ability to learn without being explicitly programmed.” During his time at IBM, Samuel developed a checkers program, which was one of the world’s first uses of self-learning. He had the program play thousands of games with itself to learn the most favorable board positions that can lead to a win. Later, with the growth of ideas like pattern recognition, many algorithms for machine learning saw significant advancements. The nearest neighbor algorithm, deep learning, support-vector machine, and boosting algorithms have all been fundamental to the evolution of machine learning. Today, machine learning includes supervised and unsupervised learning and has widely been applied to a wide variety of clustering, classification and regression problems. It is used for speech and facial recognition, fraud detection, product recommendations, dynamic pricing, and more.
In the field of healthcare and medicine, machine learning can be beneficial in many areas, including biomedical data management, automation of diagnoses, and biomarker discovery. At Cofactor, we see the biggest opportunity in biomarker discovery, specifically, building better biomarkers to predict a patient’s response to therapy. While there’s been a 500% increase in innovative new medicines developed in the last decade, we are using diagnostic approaches first developed in the 1920’s (100 years ago) to try and predict which medicines will work in which patients. Past diagnostic approaches are failing because they fail to capture the complexity of disease.
The first challenge for building better biomarkers is making sense of big data from a biological system like the human body. There are many diverse sources of data like genome-, transcriptome-, proteome-, metabolome-, epigenome-, and metagenome-level. The current method of applying machine learning to biomarker discovery is making feature selections across multiple levels of omics data that predict outcomes between different cohorts.
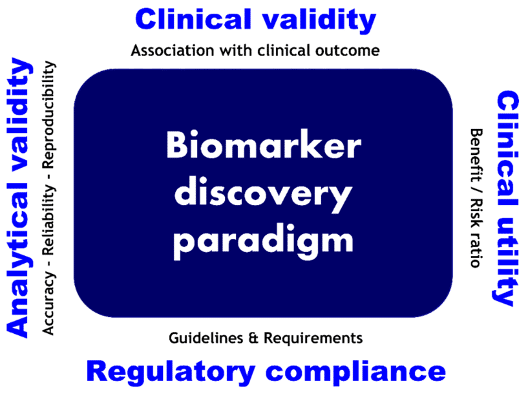
Fig 1. Biomarker development pipeline milestones (from Tebani et al., 2016)
Four pillars of a biomarker discovery pipeline (figure 1) are: analytical validity, clinical validity, clinical utility, and regulatory and ethical compliance.² Analytical validation is the key to building classifiers for effective medical decision-making. Due to the high dimensionality and system complexity of the omics data, machine learning is often used to interpret the data. The “black box” of machine learning is the predictive computational models and their validation. With a collected training dataset, certain signals or features of interests are selected. A multivariate predictive model is then built using the training dataset. Then, a validation dataset is used as input for the model to assess the performance.
When utilizing machine learning, it’s important to understand and incorporate these three points:³
- Transparency: the computational model for decision making should be clear and easy to communicate.
- Explainability: there should be an understanding of the reasoning that corresponds with each decision.
- Provability: There should be mathematical certainty behind each decision.
There should be answers to the following questions: which algorithm was used to build the model? What features are selected? What is the confidence level associated with a certain decision? Do any errors exist? What is the optimal iteration or are there other parameters to set? The computational model is not an explainable model unless all of these uncertainties are addressed.
At Cofactor Genomics, we leveraged the power of machine learning and RNA to build Health Expression Models that capture complex biology and enable quantification in heterogenous tissue samples. To align with our current clinical focus, the database contains models of key immune cells known to play a role in immune-oncology. Using machine learning to build precision diagnostics will change the future of precision medicine, allowing the right patient to receive the right treatment at the right time.
References:
1. Strimbu, K., and Tavel, J. A. (2010). What are Biomarkers? Curr Opin HIV AIDS. 2010 Nov; 5(6): 463–466. doi: 10.1097/COH.0b013e32833ed177
2. Tebani, A., Afonso, C., Marret, S., and Bekri, S. (2016). Omics-Based strategies in precision medicine: toward a paradigm shift in inborn errors of metabolism investigations. Int. J. Mol. Sci. 17. doi: 10.3390/ijms17091555
3. PwC. (2018). 2018 AI predictions. 8 insights to shape business strategy. http://pwc.com/us/AI2018